GrAPL 2021: Workshop on Graphs, Architectures, Programming, and Learning
Virtual
17 May 2021
Scope and Goals:
GrAPL is the result of the combination of two IPDPS workshops
- GABB: Graph Algorithms Building Block
- GraML: Workshop on The Intersection of Graph Algorithms and Machine Learning
Data analytics is one of the fastest growing segments of computer science. Much of the recent focus in Data Analytics has emphasized machine learning. This is understandable given the success of deep learning over the last decade. However, many real-world analytic workloads are a mix of graph and machine learning methods. Graphs play an important role in the synthesis and analysis of relationships and organizational structures, furthering the ability of machine-learning methods to identify signature features. Given the difference in the parallel execution models of graph algorithms and machine learning methods, current tools, runtime systems, and architectures do not deliver consistently good performance across data analysis workflows. In this workshop we are interested in Graphs, how their synthesis (representation) and analysis is supported in hardware and software, and the ways graph algorithms interact with machine learning. The workshop’s scope is broad which is a natural outgrowth of the wide range of methods used in large-scale data analytics workflows.
This workshop seeks papers on the theory, model-based analysis, simulation, and analysis of operational data for graph analytics and related machine learning applications. We are particularly interested in papers that:
- Provide tractability performance analysis in terms of complexity, time-to-solution, problem size, and quality of solution for systems that deal with mixed data analytics workflows.
- Discuss the problem domains and problems addressable with graph methods, machine learning methods, or both;
- Discuss programming models and associated frameworks such as Pregel, Galois, Boost, GraphBLAS, GraphChi, etc., for building large multi-attributed graphs;
- Discuss how frameworks for building graph algorithms interact with those for building machine learning algorithms;
- Discuss hardware platforms specialized for addressing large, dynamic, multi-attributed graphs and associated machine learning;
Besides regular papers, short papers (up to four pages) describing work-in-progress or incomplete but sound, innovative ideas related to the workshop theme are also encouraged.
Location:
This workshop is co-located with IPDPS 2021, held 17-21 May 2021, virtual. Registration information for IPDPS2021 can be found at here.
Program:
Keynote Talk 1:
Sparse Adjacency Matrices at the Core of Graph Databases:
GraphBLAS the Engine Behind RedisGraph Property Graph Database
Roi Lipman (RedisLabs, USA)
In the last couple of years, we have seen a rise in the number of new graph database vendors. What used to be a field with just a handful of key players, has transitioned into a vibrant arena full of innovation and competition, where performance matters most. Engineers and researcher are always trying to improve and come up with new techniques to perform graph traversals and other types of graph analysis. With the quite recent release of GraphBLAS (an open effort to define standard building blocks for graph algorithms in the language of linear algebra), we are able to define graphs using sparse adjacency matrices and evaluate queries by using linear algebra operations. RedisGraph, a property graph database, is the first to do this. Transitioning from a vertex centric point of view proved to be challenging but well worth it, as we are able to incorporate years of research and development in the graph DB world. Projects such as LAGraph (a collection of algorithms that use the GraphBLAS) can be incorporated and exposed easily to end users, and soon enough running graph queries on GPUs. GraphBLAS has indeed performed a revolution in this field. In this talk, I will present RedisGraph and the way it uses GraphBLAS to answer graph queries formulated in the Cypher query language (one of the most popular graph query languages). I'll touch on the pros and cons of using sparse adjacency matrices at the core of this DB, and a few of the classical graph algorithms (implemented by linear algebra operations) incorporated in RedisGraph.
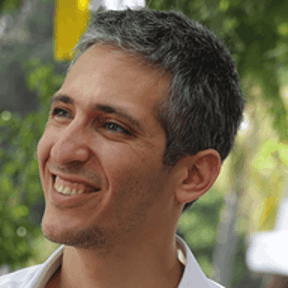
Roi Lipman is the creator of the only Linear Algebra formulation-based Graph Database at RedisLabs where he leads the development of RedisGraph since 2017. His key interests include: GraphBLAS, database systems, high performance computing, and parallel and distributed algorithms.
Keynote Talk 2:
Label Propagation and Graph Neural Networks
Austin Benson (Cornell University, USA)
Semi-supervised learning on graphs is a widely applicable problem in network science and machine learning. Two standard algorithms -- label propagation and graph neural networks -- both operate by repeatedly passing information along edges, the former by passing labels and the latter by passing node features, modulated by neural networks. These two types of algorithms have largely developed separately, and there is little understanding about their relationship and how the approaches can be meaningfully combined. In this talk, I will present some probabilistic models that unify these algorithms, showing how label propagation and graph neural network ideas are naturally connected and how this leads to algorithms that can use both effectively. The talk will also discuss computational and machine learning tradeoffs of complex, highly expressive models that are expensive to train and difficult to implement, compared to simpler, less expressive models that run faster, are easy to implement, and offer more opportunities for parallelism.
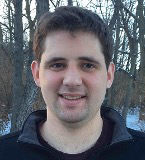
Austin Benson is an Assistant Professor of Computer Science and a Field Member of Applied Mathematics at Cornell University. His research develops numerical methods and algorithmic frameworks that enable new, better, and bigger analyses of complex network data. Austin’s research has appeared in Science, the Proceedings of the National Academy of Sciences, and SIAM Review, and has been recognized with a KDD best paper award and the Gene Golub doctoral Dissertation Award. Before joining Cornell, he received his PhD in computational and mathematical engineering at Stanford University.
Time | Event |
---|
8:00-8:05 | Welcome and Introduction |
8:05~9:55 8:05~8:50 8:50 - 9:55 |
Session 1: HPC, GraphBLAS, Tools Keynote 1 Sparse Adjacency Matrices at the Core of Graph Databases: GraphBLAS the Engine Behind RedisGraph Property Graph Database Roi Lipman (Redis Labs) LAGraph: Linear Algebra, Network Analysis Libraries, and the Study of Graph Algorithms [slide] [paper] Gábor Szárnyas (CWI Amsterdam), David A. Bader (New Jersey Institute of Technology), Timothy A. Davis (Texas A&M), James Kitchen (Anaconda), Timothy G. Mattson (Intel), Scott McMillan (SEI, Carnegie Mellon), Erik Welch (Anaconda) Introduction to GraphBLAS 2.0 [slide] [paper] Benjamin A. Brock (UC Berkeley), Aydın Buluç (LBNL, UC Berkeley), Timothy G. Mattson (Intel), Scott McMillan (SEI, Carnegie Mellon), José E. Moreira (IBM) Mathematics of Digital Hyperspace [slide] [paper] Jeremy Kepner (MIT Lincoln Laboratory), Timothy Davis (Texas A&M University), Vijay Gadepally (MIT Lincoln Laboratory), Hayden Jananthan (MIT Lincoln Laboratory, Vanderbilt), Lauren Milechin (MIT) SPbLA: The Library of GPGPU-Powered Sparse Boolean Linear Algebra Operations [slide] [paper] Egor Orachev (St. Petersburg St. Univ., JetBrains Research), Maria Karpenko (ITMO Univ.), Artem Khoroshev (BIOCAD), Semyon Grigorev (St. Petersburg St. Univ, JetBrains Research) PIGO: A Parallel Graph Input/Output Library [slide] [paper] Kasimir Gabert (Georgia Tech), Ümit V. Çatalyürek (Georgia Tech) |
9:55~10:15 | Break |
10:00~12:00 10:15~11:00 11:00 - 11:55 |
Session 2: Graph Machine Learning, Models and Applications Keynote 2 Label Propagation and Graph Neural Networks Austin Benson (Cornell University) Hybrid Power-Law Models of Network Traffic [slide] [paper] Pat Devlin (Yale), Jeremy Kepner (MIT), Ashley Luo (MIT), Erin Meger (Univ. du Québec à Montréal) Characterizing Job-Task Dependency in Cloud Workloads Using Graph Learning [slide] [paper] Zhaochen Gu (Univ. N. Texas), Sihai Tang (Univ. N. Texas), Beilei Jiang (Univ. N. Texas), Song Huang (Allstate), Qiang Guan (Kent State), Song Fu (Univ. N. Texas) Co-design of Advanced Architectures for Graph Analytics using Machine Learning [slide] [paper] Kuldeep Kurte (ORNL), Neena Imam (ORNL), Ramakrishnan Kannan (ORNL), S. M. Shamimul Hasan (ORNL), Srikanth Yoginath (ORNL) Sparse Binary Matrix-Vector Multiplication on Neuromorphic Computers [slide] [paper] Catherine D. Schuman (ORNL), Bill Kay (ORNL), Prasanna Date (ORNL), Ramakrishnan Kannan (ORNL), Piyush Sao (ORNL), Thomas E. Potok (ORNL) |
11:55~12:15 | Community Open Discussion |
Details and Dates
Due to the perduring pandemic situation, IPDPS 2021 and its workshops will be held virtually.
The GrAPL organizing committee has planned an exciting online program, consisting in two LIVE 120-minute sessions on May 17 (starting at 8:00 AM PDT, 3:00 PM UTC, 5:00 PM CET) with keynotes and live Q&A for each accepted paper. The schedule below contains links to the abstracts of the keynote talks and to 3 minutes lighting talk videos (available on or before May 14) of accepted papers pitching the GrAPL community to read the papers and prepare to ask questions at the online sessions.
Register at the IPDPS website to get instructions on how to access papers and static presentations for GrAPL: http://www.ipdps.org
To attend the Zoom Sessions, we ask participants to watch the videos, read the papers, prepare questions, and register in advance at the following link: https://tinyurl.com/GrAPL-2021-Registration
The organizing committee will then provide the link to the session.
Note: to access the papers, user id and password, provided to registered IPDPS attendees, are required.
Workshop Organizers:
General Co-Chairs:
- Scott McMillan (CMU SEI)
- Manoj Kumar (IBM)
Program Chair:
- Nesreen Ahmed (Intel)
GrAPL's Little Helpers:
- Tim Mattson (Intel)
- Antonino Tumeo (PNNL)
Technical Program committee members (in addition to the chair):
- Paul Bogdan, University of Southern California , US
- Anu Bourgeois, Georgia State University , US
- Aydin Buluç, Lawrence Berkeley National Laboratory; University of California, Berkeley, US
- John Gilbert, University of California, Santa Barbara, US
- Sergio Gomez, Universitat Rovira i Virgili , ES
- Stratis Ioannidis, Northeastern University, US
- Kamesh Madduri, Pennsylvania State University , US
- Hesham Mostafa, Intel Labs, US
- Robert Rallo, Pacific Northwest National Laboratory, US
- Indranil Roy, Natural Intelligence Systems, Inc. , US
- Ponnuswamy Sadayappan, University of Utah; Pacific Northwest National Laboratory, US
- Shaden Smith, Microsoft Corporation, US
- Yizhou Sun, University of California, Los Angeles, US
- Ramachandran Vaidyanathan, Louisiana State University , US
- Alexander van der Grinten, Humboldt-University of Berlin , DE
- Flavio Vella, Free University of Bozen, IT
Steering committee:
- David A. Bader (New Jersey Institute of Technology)
- Aydın Buluç (LBNL)
- John Feo (PNNL)
- John Gilbert (UC Santa Barbara)
- Mahantesh Halappanavar (PNNL)
- Tim Mattson (Intel)
- Ananth Kalyanaraman (Washington State University)
- Jeremy Kepner (MIT Lincoln Labs)
- Danai Koutra (University of Michigan)
- Antonino Tumeo (PNNL)